Data Responsibility With Great Data Comes Great Responsibility
With great amounts of data comes great responsibility. This isn’t just a catchy phrase; it’s a crucial concept in today’s data-driven world. Vast datasets hold immense potential for good, but their misuse can have devastating consequences. From ethical implications to societal impact, this exploration dives deep into the multifaceted nature of data responsibility, examining how we can harness the power of data while mitigating the risks.
This discussion will unpack the responsibilities associated with large datasets, including the ethical considerations, potential biases, and the importance of transparency and accountability. We’ll delve into the intricacies of data governance, lifecycle management, and security, offering practical insights for organizations and individuals navigating the data landscape. We’ll also look at real-world examples of both successes and failures in data handling, demonstrating the crucial role of human judgment in this complex field.
The Nature of Data Responsibility: With Great Amounts Of Data Comes Great Responsibility
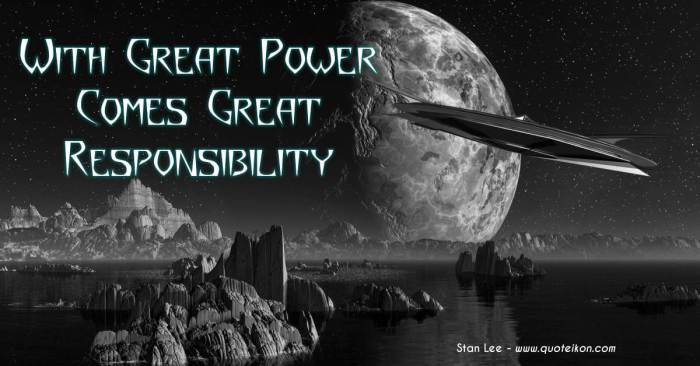
The sheer volume of data generated daily demands a new level of ethical and practical consideration. We are entering an era where access to massive datasets grants unprecedented power, but with that power comes a profound responsibility to use it wisely and ethically. The ability to shape narratives, influence decisions, and even predict future trends through data analysis necessitates a meticulous understanding of its implications.
This responsibility transcends mere technical proficiency; it encompasses a profound understanding of societal impact, ethical considerations, and the potential for misuse.The ‘great responsibility’ inherent in large datasets stems from the potential for significant harm if handled carelessly. A flawed algorithm or biased dataset can perpetuate harmful stereotypes, lead to discriminatory practices, or result in catastrophic miscalculations in critical sectors like healthcare or finance.
We often hear about “with great amounts of data comes great responsibility,” and it’s a powerful concept. This rings especially true when considering advancements in reproductive technology, like the recent “calif s fertility flap and the future of reproductive tech” debate. This flap highlights the ethical dilemmas surrounding the use of personal data in fertility treatments, and the potential for misuse.
Ultimately, responsible data handling in reproductive technology is crucial for ensuring ethical and equitable access to these vital services, ensuring that with great data comes great responsibility.
Imagine a loan application algorithm inadvertently discriminating against a particular demographic, or a self-driving car system making a life-or-death decision based on faulty data. These are not hypothetical scenarios; such incidents have occurred and highlight the need for stringent oversight and ethical guidelines.
Different Approaches to Data Governance
Various approaches to data governance exist, each with its own strengths and weaknesses. Centralized systems often offer greater control and standardization but may stifle innovation and agility. Decentralized models, on the other hand, encourage quicker adaptation but can lead to inconsistencies and data silos. Hybrid approaches aim to strike a balance between these extremes, offering a tailored solution for specific organizational needs.
The optimal approach depends on the size and complexity of the dataset, the industry’s regulatory environment, and the organization’s internal structure.
Ethical Implications of Wielding Data Power
The ability to analyze and interpret vast datasets carries significant ethical responsibilities. Data-driven decisions can have far-reaching consequences, affecting individuals’ lives, impacting communities, and shaping societal norms. Therefore, ensuring data integrity, minimizing bias, and maintaining transparency are paramount. Maintaining accurate and unbiased datasets is critical for building trust and preventing harm. Organizations must adopt transparent data collection and analysis practices, ensuring accountability for decisions based on data insights.
Perspectives on Data Integrity
Data integrity is crucial for the reliability of any analysis and decision-making process. Different stakeholders view data integrity from various perspectives. From a business perspective, maintaining accurate and consistent data is essential for effective operations and achieving strategic goals. A regulatory perspective emphasizes compliance with data protection laws and standards. An ethical perspective focuses on the moral obligations to avoid bias, maintain privacy, and use data responsibly.
Risks Associated with Unchecked Data Access
Unchecked data access can lead to various risks, ranging from privacy violations to potential misuse of sensitive information. Unauthorized access or breaches can expose confidential data, leading to reputational damage and legal liabilities. Furthermore, the misuse of data for malicious purposes, such as targeted advertising or manipulation of public opinion, can have severe social and political consequences. Robust security measures, access controls, and clear data usage policies are essential to mitigate these risks.
Data Privacy in the Face of Massive Datasets
Protecting data privacy is paramount in the era of massive datasets. Individuals must have control over their personal data and be aware of how it is collected, used, and shared. Data anonymization, encryption, and access controls are crucial for safeguarding sensitive information. Organizations must prioritize transparency in data practices and comply with relevant data privacy regulations.
A Framework for Responsible Data Handling, With great amounts of data comes great responsibility
A framework for responsible data handling in organizations requires a multi-faceted approach. It should encompass clear data governance policies, including data collection, storage, usage, and disposal guidelines. Establishing a dedicated data ethics committee can ensure continuous oversight and ethical considerations in all data-related activities. Regular audits and compliance checks are necessary to maintain adherence to standards. A robust training program for employees on data ethics and responsible data handling is essential to foster a culture of accountability and awareness.
Key Principles for Ethical Data Usage
Data ethics should underpin all data-related activities. Transparency, accountability, and fairness are essential principles. Data should be collected and used ethically, minimizing potential harm and maximizing benefit. Privacy, security, and integrity are foundational elements. Bias detection and mitigation are crucial steps to ensure fairness and equity.
The principle of consent is paramount, ensuring individuals are informed and have control over their data. Organizations should actively seek input from diverse perspectives to ensure data use reflects a range of needs and values.
Data Handling and its Impact
Data, in its vast and ever-growing form, has become an indispensable resource in the modern world. From personal preferences to global trends, data underpins our understanding and shapes our decisions. However, the power that comes with data also carries a significant responsibility. The manner in which we handle data profoundly impacts society, demanding careful consideration of both the potential benefits and the inherent risks.The potential societal impact of data-driven decisions is profound and multifaceted.
Algorithms trained on vast datasets can influence everything from loan applications to criminal justice assessments. The accuracy and fairness of these systems are critical, as biased data can lead to discriminatory outcomes. Furthermore, the lack of transparency in how these systems operate can erode public trust and hinder accountability. This necessitates a careful and critical evaluation of the potential consequences before implementing data-driven solutions.
Societal Impact of Data-Driven Decisions
Data-driven decisions have a significant impact on societal well-being. Properly utilized, data can empower communities, inform policy, and drive innovation. However, flawed or biased data can lead to unfair or discriminatory outcomes, potentially exacerbating existing societal inequalities. For example, a loan application algorithm trained on historical data that reflects societal biases might perpetuate financial disparities. The responsibility for ensuring fairness and equity in data-driven decision-making rests heavily on those who design and deploy these systems.
Potential Benefits and Drawbacks of Data-Intensive Technologies
Data-intensive technologies, like machine learning and artificial intelligence, offer tremendous potential for advancements in various fields. They can optimize processes, personalize experiences, and drive scientific discoveries. However, these technologies are not without their drawbacks. Potential risks include data breaches, algorithmic bias, and the displacement of human labor. These potential drawbacks necessitate a comprehensive understanding of the trade-offs and a proactive approach to mitigating the risks.
Bias in Data and Unfair Outcomes
Bias in data can lead to unfair outcomes in various sectors. Algorithms trained on biased datasets can perpetuate and even amplify existing societal biases. For example, facial recognition systems trained primarily on images of light-skinned individuals may perform less accurately on images of people with darker skin tones. Understanding and addressing these biases is crucial to ensure fairness and equity in data-driven decision-making.
Data for Positive Change
Data can be a powerful tool for promoting positive change. For instance, data analysis can identify patterns of crime, enabling law enforcement to allocate resources more effectively. Similarly, data can inform public health initiatives, leading to improved disease prevention and treatment. The key lies in using data ethically and transparently.
Importance of Transparency in Data Practices
Transparency in data practices is essential for building trust and accountability. Individuals should understand how their data is being collected, used, and shared. Clear data policies and procedures foster public confidence and reduce the potential for misuse. Transparency is paramount to maintaining public trust and enabling informed consent.
It’s a truism that with great amounts of data comes great responsibility. Think about TomTom’s recent move to integrate live services into their Navvy app; tomtom adds live services to navvy This means their navigation data is constantly updated, offering real-time traffic and route adjustments. However, with this increased reliance on live data, ensuring accuracy and user privacy becomes paramount.
Ultimately, handling massive datasets requires a strong ethical framework and a commitment to responsible use.
Methods for Mitigating Data Misuse
Various methods can mitigate the risks associated with data misuse. These include robust data security protocols, ethical guidelines for data collection and analysis, and regulations to ensure accountability. The development of clear and enforceable data governance policies is also crucial. Implementing these measures can significantly reduce the risks of data misuse.
We’ve all heard the saying, “with great amounts of data comes great responsibility.” This rings true for tech updates like Ford’s Sync update, which now includes fuel-saving tips in navigation. The new feature, found in Ford’s Sync update gives directions fuel saving tips , is a smart way to use data for a better experience. Ultimately, responsible data usage in tech is key to its continued positive impact on our lives.
Case Studies of Poor Data Handling
Several organizations have faced consequences due to poor data handling. Examples include instances of data breaches that exposed sensitive information, leading to significant financial and reputational damage. Understanding the consequences of poor data handling can inform best practices and promote responsible data management.
Data Lifecycle Management
Stage | Description | Activities | Key Considerations |
---|---|---|---|
Data Collection | Gathering data from various sources | Defining data requirements, selecting appropriate data sources, establishing data quality standards | Data accuracy, completeness, consistency, and relevance |
Data Storage | Preserving data for future use | Choosing suitable storage solutions, ensuring data security, establishing backup and recovery plans | Data integrity, accessibility, and availability |
Data Processing | Transforming and analyzing data | Data cleaning, transformation, and integration, developing analytical models | Data quality, consistency, and integrity throughout processing |
Data Utilization | Using data for decision-making | Interpreting data insights, communicating findings, implementing data-driven strategies | Data-driven decision making, ethical implications, and transparency |
Ensuring Data Security in a Large-Scale Environment
Ensuring data security in a large-scale environment requires a multifaceted approach. Robust encryption techniques, access controls, and regular security audits are essential. Additionally, fostering a culture of security awareness among employees is crucial. This comprehensive approach helps protect sensitive information from unauthorized access and misuse.
Data and Accountability
Data, in its sheer volume and complexity, demands a strong framework of accountability. Organizations that leverage data must be transparent and responsible in their collection, usage, and storage. This is crucial not just for maintaining trust but also for ensuring ethical and effective decision-making. Accountability is paramount to avoiding potential harm and maximizing the positive impact of data.The very nature of data-driven decision-making necessitates a clear understanding of who is responsible for the outcomes.
This extends beyond just the technical aspects of data management to encompass ethical considerations, legal compliance, and the potential social impact of data-driven products and services. A robust framework for data accountability is essential to mitigate risks and build public trust.
Importance of Accountability in Data-Driven Organizations
Accountability in data-driven organizations fosters trust and ensures ethical practices. It Artikels clear responsibilities for data handling and decision-making, creating a system where individuals and teams are answerable for their actions. This is essential for building a culture of integrity and preventing potential misuse or harm.
Examples of How Individuals Can Hold Organizations Accountable
Individuals can hold organizations accountable for their data practices through various avenues. Direct communication with the organization, filing formal complaints with regulatory bodies, and engaging in public discourse are effective strategies. Supporting organizations that prioritize ethical data practices and boycotting those that demonstrate irresponsibility are other powerful tools. Furthermore, individuals can utilize data transparency tools to monitor data collection and usage.
Legal and Regulatory Frameworks Surrounding Data Usage
Legal and regulatory frameworks, like GDPR in Europe and CCPA in California, establish guidelines for data collection, storage, and use. These regulations aim to protect individuals’ privacy rights and ensure organizations adhere to ethical standards. Understanding and complying with these regulations is crucial for organizations operating in various jurisdictions.
Role of Data Ethics Committees in Ensuring Responsible Data Practices
Data ethics committees play a vital role in guiding organizations towards responsible data practices. They provide a forum for discussing ethical dilemmas, reviewing data policies, and ensuring compliance with relevant regulations. Their presence promotes transparency and mitigates potential risks associated with data usage.
Potential for Public Backlash Against Irresponsible Data Practices
Irresponsible data practices can lead to significant public backlash. Negative publicity, boycotts, and legal challenges are just a few examples of the consequences organizations face when they disregard ethical considerations and user privacy. This underlines the importance of proactively addressing data privacy and accountability concerns.
Comparison of Different Models of Data Governance and Accountability
Various models for data governance and accountability exist, each with its strengths and weaknesses. Centralized models offer a high degree of control but can be slow and inflexible. Decentralized models, on the other hand, offer greater agility but require careful coordination. Hybrid models combine aspects of both approaches, attempting to balance control and flexibility. Understanding the characteristics of each model is crucial in selecting the appropriate approach for a given organization.
Examples of Successful Data Governance Initiatives
Numerous organizations have implemented successful data governance initiatives. These initiatives often involve establishing clear data ownership policies, implementing robust data security measures, and fostering a culture of data ethics. Examples include companies that have proactively addressed data breaches, effectively managed personal data, and established transparent data policies.
Data Privacy Regulations Comparison
Regulation | Jurisdiction | Key Principles | Enforcement Mechanisms |
---|---|---|---|
GDPR | European Union | Data minimization, purpose limitation, and data subject rights | Fines, investigations, and injunctions |
CCPA | California, USA | Individual control over personal data, right to deletion, and opt-out rights | Enforcement by the California Attorney General |
HIPAA | United States | Protection of health information, confidentiality, and security | Enforcement by the Department of Health and Human Services |
PIPEDA | Canada | Protection of personal information, transparency, and accountability | Enforcement by the Office of the Privacy Commissioner of Canada |
Building a Data Governance Policy
A data governance policy is a crucial document outlining how an organization handles and manages its data. The process involves defining clear data ownership and responsibility, establishing data quality standards, and outlining procedures for data security and privacy. Crucially, it must address compliance with relevant regulations. A well-defined policy is essential for maintaining trust, preventing misuse, and ensuring the ethical use of data.
Data-Driven Decision Making
Data-driven decision-making has become increasingly prevalent across various sectors. Leveraging data to inform choices allows for a more objective and potentially more effective approach compared to relying solely on intuition or experience. However, this process is not without its complexities, especially concerning potential biases. Understanding these biases, developing strategies to mitigate them, and incorporating diverse perspectives are crucial for ensuring that data-driven decisions lead to equitable and beneficial outcomes.
Potential for Bias in Data-Driven Decision-Making
Data sets often reflect existing societal biases, whether intentionally or unintentionally. These biases can manifest in various forms, such as gender, racial, or socioeconomic disparities. If these biases are not recognized and addressed during the data analysis process, they can be inadvertently amplified in the resulting decisions. For instance, a hiring algorithm trained on historical data that reflects gender stereotypes might perpetuate those stereotypes in its hiring recommendations.
Methods for Mitigating Bias in Data Analysis
Various methods can help mitigate bias in data analysis. These include: careful data collection practices, ensuring data representation is balanced, employing techniques like stratified sampling, and implementing checks for bias throughout the analysis process. Bias detection tools and regular audits can also help to identify and correct biases in data sets. Regular evaluation and review of algorithms and models for potential bias are essential for ensuring fairness and accuracy.
Importance of Diverse Perspectives in Data Interpretation
Data interpretation is inherently subjective. Incorporating diverse perspectives during the data interpretation phase is critical. Individuals from various backgrounds bring unique experiences, insights, and cultural contexts that can challenge assumptions and reveal potential biases in the data. This leads to a more comprehensive understanding of the data and more robust decisions. A team of analysts with varying viewpoints can uncover blind spots in the data and provide more nuanced interpretations.
Examples of Data-Driven Decision-Making Improvements
Data-driven approaches can significantly enhance decision-making across diverse fields. In healthcare, data analysis can identify patterns in patient demographics and disease progression to personalize treatment plans. In retail, data on customer preferences and purchasing patterns can be used to optimize inventory management and marketing strategies. In finance, data analysis can identify investment opportunities and mitigate risks.
Role of Human Judgment in Data-Driven Decision-Making
Data analysis should not replace human judgment entirely. Humans are essential in contextualizing data, identifying anomalies, and making ethical considerations that data alone may not capture. Human judgment plays a crucial role in assessing the broader implications of data-driven decisions and in ensuring that the decisions align with societal values and ethical principles. Data should be viewed as a tool to support, not replace, human intuition and critical thinking.
Potential for Algorithmic Bias to Create Unfair Outcomes
Algorithmic bias can lead to unfair outcomes in areas such as loan applications, criminal justice, and hiring. If algorithms are trained on biased data, they can perpetuate and amplify existing inequalities. It is crucial to recognize and address algorithmic bias to ensure fair and equitable outcomes. Techniques like fairness-aware algorithms and ongoing monitoring can help mitigate algorithmic bias.
Data-Driven Decision-Making Framework
Step | Description | Example | Potential Pitfalls |
---|---|---|---|
Data Collection | Gathering relevant and unbiased data from reliable sources. | Collecting customer feedback through surveys and analyzing website traffic data. | Using biased sampling methods, collecting incomplete data, or relying on outdated data. |
Data Analysis | Identifying patterns, trends, and insights from the collected data. | Analyzing sales data to identify seasonal trends and customer preferences. | Incorrectly interpreting data, overlooking outliers, or oversimplifying complex relationships. |
Decision Making | Formulating decisions based on data insights, considering ethical implications, and involving diverse perspectives. | Adjusting marketing strategies based on customer feedback and sales trends. | Over-reliance on data, ignoring contextual factors, and failing to incorporate human judgment. |
Evaluation and Feedback | Assessing the effectiveness of decisions and making adjustments to future strategies. | Evaluating the impact of marketing campaigns on sales and customer engagement. | Ignoring negative feedback, failing to adapt to changing circumstances, or underestimating the impact of unforeseen factors. |
Avoiding Over-Reliance on Data-Driven Insights
Data-driven insights should be used as a tool to enhance decision-making, not as the sole determinant. Considering the broader context, ethical implications, and human judgment is crucial. Over-reliance can lead to overlooking important factors not captured in the data, potentially leading to poor or harmful decisions. It is essential to maintain a balance between data-driven insights and human judgment.
Questions for Evaluating Data-Driven Decisions
- Does the decision consider the potential impact on diverse groups and individuals?
- Has the decision process been transparent and inclusive?
- Have potential biases in the data been identified and addressed?
- Has the decision been evaluated for its ethical implications?
- Does the decision consider the broader context beyond the data itself?
Illustrative Examples
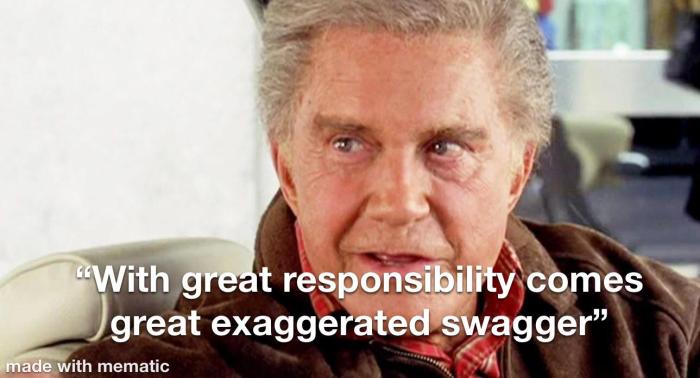
Data, in its raw form, is a powerful tool. However, its potential for both good and harm is undeniable. Understanding how data has been used – both effectively and ineffectively – provides critical insights into responsible data practices. This section explores real-world examples to illuminate the complexities of data handling.
Historical Case Study of Data Misuse: The Tuskegee Syphilis Study
The Tuskegee Syphilis Study, conducted by the U.S. Public Health Service from 1932 to 1972, stands as a chilling example of data misuse and unethical research practices. Researchers deliberately withheld treatment for syphilis in African American men, observing the progression of the disease without their knowledge or consent. This study violated fundamental ethical principles of informed consent, beneficence, and respect for persons, resulting in severe harm to the participants and lasting mistrust in medical research.
The study’s profound impact underscored the need for ethical oversight in research and the protection of vulnerable populations. This case exposed the devastating consequences of prioritizing data collection over the well-being of research subjects.
Modern Example of Ethical Data Handling: The use of AI in Healthcare
Modern healthcare increasingly leverages artificial intelligence (AI) to analyze patient data for improved diagnosis and treatment. AI algorithms can identify patterns and anomalies in medical images, predict patient risks, and personalize treatment plans. For instance, AI can assist in early detection of diseases like cancer, leading to timely intervention and improved outcomes. The ethical use of AI in healthcare involves stringent data privacy and security protocols, ensuring patient consent and transparency in the use of their data.
Examples of ethical AI applications include the development of algorithms trained on anonymized patient data to improve diagnostic accuracy while adhering to strict privacy regulations. Data is used responsibly when it’s leveraged to benefit patients and improve health outcomes, while maintaining patient confidentiality and rights.
Scenario of Data Bias Leading to Negative Outcomes: Predictive Policing
Predictive policing algorithms, designed to predict crime hotspots, can inadvertently perpetuate existing societal biases. If these algorithms are trained on data reflecting historical biases, such as racial profiling, they may amplify these biases in their predictions. This can lead to increased surveillance and police activity in communities already disproportionately affected by crime, further exacerbating existing inequalities. For example, if the data used to train a predictive policing algorithm reflects a higher crime rate in certain neighborhoods based on demographic characteristics, the algorithm may misinterpret this as a higher likelihood of crime in that area, thus leading to more policing in the area, potentially leading to negative interactions with law enforcement.
The crucial point is to understand how bias in data can lead to disproportionate impacts on certain communities.
Case Study of Responsible Data Practices Fostering Trust: Open Data Initiatives
Open data initiatives, where governments and organizations release datasets for public use, foster transparency and trust. By making data accessible, open data empowers citizens to analyze trends, hold institutions accountable, and engage in informed decision-making. For instance, open government data can be used to monitor environmental conditions, analyze public health trends, or assess infrastructure needs. The transparency provided by open data creates trust between the public and institutions.
Such initiatives demonstrate that data sharing can strengthen democracy and promote societal well-being.
Using Data to Create Positive Social Change: Education Data Analytics
Education data analytics can identify patterns in student performance and resource allocation, enabling educators to tailor interventions and improve learning outcomes. Data analysis can reveal disparities in access to resources, identify struggling students, and highlight areas requiring targeted support. For instance, data can reveal patterns in student attendance, which can help educators understand reasons for poor attendance and devise strategies to improve student engagement and retention.
Data-driven strategies can ensure equitable access to education for all students.
Ethical Dilemmas Related to Data Collection and Use
- Balancing the need for data with individual privacy rights.
- Ensuring data accuracy and avoiding bias in data collection.
- Protecting sensitive data from unauthorized access and breaches.
- Establishing clear guidelines for data ownership and usage.
- Accountability for data breaches and misuse.
These ethical dilemmas are central to responsible data practices, highlighting the importance of balancing the benefits of data with the protection of individual rights.
Best Practices for Building Data Literacy
- Understanding the limitations of data.
- Critically evaluating the source of data.
- Developing critical thinking skills to analyze data effectively.
- Promoting collaboration between data experts and non-experts.
- Fostering a culture of data literacy.
Data literacy equips individuals with the skills to interpret, evaluate, and use data responsibly. By fostering data literacy, we empower individuals to make informed decisions and hold institutions accountable.
Data Security Breach and its Consequences: Equifax Data Breach
The 2017 Equifax data breach exposed the personal information of over 147 million people, highlighting the vulnerabilities of large data systems. The breach exposed sensitive data, including names, addresses, social security numbers, and credit card information. This led to significant financial losses, identity theft, and reputational damage for Equifax. The consequences demonstrated the importance of robust data security measures and the potential for widespread harm caused by data breaches.
Data-Related Regulations and Laws
- General Data Protection Regulation (GDPR)
- California Consumer Privacy Act (CCPA)
- Health Insurance Portability and Accountability Act (HIPAA)
- Children’s Online Privacy Protection Act (COPPA)
These regulations and laws are crucial for safeguarding data and promoting responsible data practices. Compliance with these regulations ensures the protection of personal data and addresses ethical considerations in data handling.
Last Point
In conclusion, the responsibility associated with data is not just about avoiding mistakes; it’s about proactively building a system where data is used ethically and responsibly. By understanding the potential pitfalls and proactively implementing robust data governance frameworks, we can harness the power of data to create a better future. The ethical use of data is not a choice but a necessity in today’s interconnected world.