Tuning Up Convergence Engine QA with Nokias IRA Frimere
Tuning up the convergence engine qa with nokias ira frimere – Tuning up the convergence engine QA with Nokia’s IRA Frimere is crucial for optimizing communication systems. This deep dive explores the intricate process of fine-tuning the convergence engine, a vital component in modern communication systems. We’ll examine how Nokia’s IRA Frimere tools enhance quality assurance (QA) procedures, highlighting best practices and potential pitfalls along the way. Understanding performance metrics and troubleshooting techniques will be paramount in achieving optimal results.
The convergence engine, often the backbone of communication networks, requires meticulous QA to ensure a seamless user experience. Nokia’s IRA Frimere offers specialized tools and techniques to analyze and improve its performance, enabling efficient troubleshooting and optimal system tuning. This exploration delves into the intricacies of convergence engine QA, leveraging Nokia’s IRA Frimere to achieve robust and reliable systems.
Introduction to Convergence Engine QA Tuning
The convergence engine is a crucial component in modern communication systems, acting as a central hub for integrating various communication channels like voice, video, and data. It seamlessly manages the flow of information between these channels, ensuring a unified user experience. Efficient and reliable operation of the convergence engine is vital for delivering high-quality services.Quality Assurance (QA) plays a critical role in ensuring software meets specified requirements and functions as intended.
For the convergence engine, meticulous QA is paramount to guarantee smooth operation, minimize errors, and ultimately provide a positive user experience. Effective tuning of the convergence engine through rigorous QA testing is essential for optimizing performance and reliability.
We’re deep in the trenches tuning up the convergence engine QA with Nokia’s IRA Frimere. It’s a meticulous process, but surprisingly, the recent shift in digital engagement, like the way YouTube ushered in change with Obama videos , has given us some fresh perspectives. Ultimately, though, we need to get back on track with the Nokia IRA Frimere tuning to ensure a seamless user experience.
Convergence Engine Function
The convergence engine’s core function is to manage the integration of different communication services. This involves routing calls, handling data transmissions, and coordinating multimedia streams. A well-functioning convergence engine provides a seamless experience for users, regardless of the communication method employed. Poorly performing engines can lead to dropped calls, delayed video streams, or corrupted data transmissions.
Significance of QA in Convergence Engine Development
Comprehensive QA testing is vital for identifying and resolving issues early in the development lifecycle. Thorough testing ensures the convergence engine meets its performance targets, handles various network conditions, and adapts to different user loads. This proactive approach prevents significant problems from arising in production, minimizing the impact on end-users.
Tuning the Convergence Engine
Tuning the convergence engine involves a systematic process of optimizing its performance and stability. This often includes:
- Performance benchmarking under various load conditions.
- Stress testing to evaluate the engine’s ability to handle peak loads and unexpected traffic spikes.
- Thorough regression testing to ensure that modifications or new features do not introduce new bugs or compromise existing functionality.
- Security testing to identify and mitigate vulnerabilities in the engine’s architecture.
These steps are crucial for achieving the desired level of reliability and performance.
Impact of Poor Convergence Engine Performance
Poor convergence engine performance can negatively affect the user experience in several ways. Users may experience dropped calls, delayed video streams, or difficulty connecting to different communication channels. This can lead to frustration, reduced productivity, and a negative perception of the overall communication system. For example, in a video conference, poor convergence engine performance might result in distorted audio, delayed video feeds, or even the complete loss of connection.
A consistent lack of smooth service can create a poor user experience.
Role of Nokia’s IRA Frimere in Convergence Engine QA
Nokia’s IRA Frimere is a critical tool for performing detailed QA on the convergence engine. It provides a comprehensive framework for testing and validating the engine’s behavior across various communication scenarios. This framework allows for simulating complex network conditions, emulating different user behaviors, and evaluating the engine’s response to various stimuli. IRA Frimere’s use significantly improves the quality and reliability of the convergence engine by identifying and resolving potential issues in a controlled environment.
Nokia IRA Frimere
Nokia’s IRA Frimere provides a comprehensive suite of tools and techniques for quality assurance (QA) of convergence engines, specifically focusing on testing and analysis within a network environment. It’s designed to simulate various network conditions and user interactions to identify potential issues and ensure the reliability and performance of the convergence engine. This allows for proactive problem identification before deployment, minimizing potential disruptions and ensuring a smooth user experience.
Tools and Techniques Offered by IRA Frimere
IRA Frimere offers a suite of tools for simulating different network conditions, including varying bandwidths, latency, and packet loss. These simulations enable comprehensive testing of the convergence engine under various stress scenarios. It also includes a powerful analysis toolset for interpreting the results of the simulated tests, helping to pinpoint specific issues and bottlenecks. These tools and techniques provide a more realistic representation of a live network environment, allowing for a more thorough evaluation of the convergence engine’s performance.
Key Functionalities of IRA Frimere, Tuning up the convergence engine qa with nokias ira frimere
IRA Frimere’s core functionalities encompass simulating network topologies, emulating user traffic patterns, and monitoring the convergence engine’s response to these simulated conditions. It allows for the creation of custom scenarios tailored to specific use cases, and it provides real-time visibility into the convergence engine’s internal workings. These functionalities facilitate a thorough understanding of the convergence engine’s behavior in various situations, ensuring its compatibility and effectiveness across different network conditions.
Furthermore, it allows for the identification of potential performance bottlenecks and resource limitations within the convergence engine.
Methodologies Used within IRA Frimere
IRA Frimere employs a variety of testing methodologies, including load testing, stress testing, and performance testing. Load testing assesses the engine’s capacity to handle a given volume of user traffic, while stress testing determines its resilience under extreme conditions. Performance testing evaluates the engine’s efficiency and responsiveness in processing various tasks. These methodologies help to identify the engine’s limitations and optimize its performance under different operational profiles.
Strengths and Weaknesses of Using IRA Frimere
IRA Frimere offers a significant advantage in providing a controlled environment for testing convergence engines. Its ability to simulate diverse network conditions allows for a thorough evaluation of the engine’s behavior under realistic stress scenarios. However, a limitation is the potential for oversimplification of real-world network complexities, as the simulated environment may not fully capture all the subtle nuances of a real network environment.
I’ve been digging into tuning up the convergence engine QA with Nokia’s IRA frimere, and it’s been a fascinating process. It’s all about optimizing the system’s performance, which, surprisingly, has some parallels with fuel efficiency strategies. For example, checking out how Ford’s Sync update gives directions fuel saving tips here shows how small tweaks can yield big results.
Ultimately, both tasks hinge on finding the most efficient path to a goal, and I’m finding the similarities quite useful as I work through the convergence engine QA.
The need for specialized expertise in configuring and interpreting results can also be a drawback.
IRA Frimere Test Scenarios for the Convergence Engine
Scenario | Description | Expected Outcome |
---|---|---|
Scenario 1: High-Load Testing | Simulate a significant increase in user traffic to evaluate the engine’s performance under heavy load. | Engine should maintain acceptable response times and stability. |
Scenario 2: Low-Bandwidth Testing | Simulate low network bandwidth conditions to assess the engine’s ability to function effectively under constrained resources. | Engine should adapt to low bandwidth without significant performance degradation. |
Scenario 3: Latency Testing | Simulate varying levels of network latency to evaluate the engine’s responsiveness to network delays. | Engine should handle network latency without compromising user experience. |
Scenario 4: Packet Loss Testing | Simulate packet loss to assess the engine’s robustness in the presence of network interruptions. | Engine should retransmit lost packets without causing significant disruption. |
Scenario 5: Multi-User Interaction Testing | Simulate concurrent user interactions to assess the engine’s ability to handle multiple simultaneous requests. | Engine should manage concurrent requests without significant performance degradation or system crashes. |
Convergence Engine Performance Metrics
Fine-tuning the convergence engine is crucial for optimal network performance, especially within Nokia’s IRA Frimere environment. Understanding the key performance indicators (KPIs) is paramount to identifying bottlenecks and areas for improvement. This section delves into the metrics used to evaluate convergence engine effectiveness and their relevance to Nokia’s IRA Frimere, providing a framework for interpreting these metrics in the context of tuning efforts and network configurations.
Key Performance Indicators (KPIs)
The effectiveness of the convergence engine is measured by several critical KPIs. These metrics provide insights into the engine’s efficiency, resource utilization, and overall performance within the network. These metrics are critical for identifying and resolving performance issues, ensuring a smooth and reliable user experience.
Convergence Engine Metrics and Units
This table Artikels various convergence engine metrics and their corresponding units, offering a standardized framework for performance analysis. Understanding these units is essential for accurately interpreting performance data and making informed decisions about tuning.
Metric | Unit | Description |
---|---|---|
Packet Loss Rate | % | Percentage of packets lost during transmission. |
Packet Delay | ms | Time taken for a packet to travel through the network. |
CPU Utilization | % | Percentage of processing power consumed by the convergence engine. |
Memory Utilization | MB | Amount of RAM used by the convergence engine. |
Throughput | Mbps | Rate at which data is transmitted through the network. |
Call Drop Rate | % | Percentage of calls dropped due to network congestion. |
Session Establishment Time | ms | Time required to establish a session. |
Interpreting Metrics for Tuning
Interpreting the metrics in the context of tuning involves analyzing their relationships and trends. For instance, a high packet loss rate might indicate network congestion or insufficient bandwidth, prompting adjustments to network configurations. Similarly, high CPU utilization could signify that the convergence engine is overloaded, requiring optimization of the algorithms or resource allocation. By tracking these metrics over time, patterns and correlations can be identified, leading to targeted tuning strategies.
Impact of Network Configurations
Different network configurations significantly impact convergence engine performance metrics. A network with a high density of devices or high traffic volume will naturally exhibit higher CPU utilization and packet loss rates compared to a less congested network. Similarly, the choice of routing protocols, QoS settings, and the overall network architecture influence the performance of the convergence engine. Understanding these relationships allows for tailored tuning strategies for specific network environments.
A network with a complex topology, utilizing multiple protocols, may demand more refined tuning strategies compared to a simpler network.
Issues and Troubleshooting
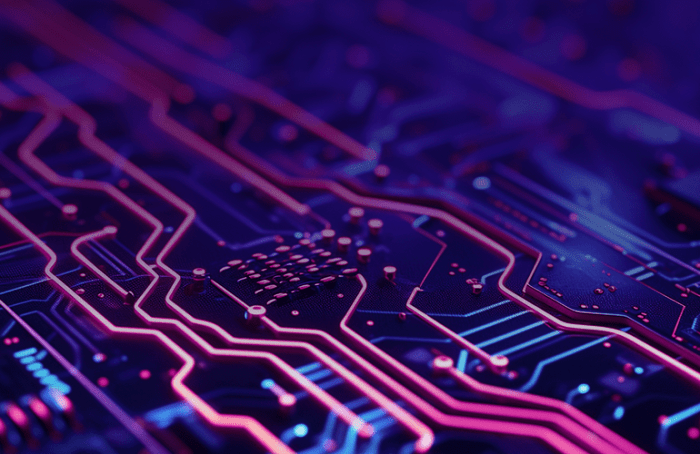
Tuning the convergence engine’s performance, especially when integrating with Nokia IRA Frimere, can encounter various challenges. Understanding these potential pitfalls and developing effective troubleshooting strategies are crucial for maintaining optimal system performance. A well-defined approach, leveraging the capabilities of IRA Frimere, is essential for identifying and resolving issues efficiently.
Common Convergence Engine Tuning Issues
Identifying potential problems proactively is key to preventing significant performance degradation. Common issues include:
- Inconsistent performance across different test scenarios:
- Unexpected drops in throughput or latency:
- Resource exhaustion (CPU, memory, disk):
- Configuration errors within the convergence engine or IRA Frimere:
- Compatibility issues between the convergence engine and other components in the system:
- Network bottlenecks impacting data transfer rates:
- Issues related to data synchronization between the convergence engine and the IRA Frimere.
Troubleshooting Strategies using IRA Frimere
IRA Frimere provides valuable insights into the convergence engine’s internal operations, enabling effective troubleshooting. Leveraging its capabilities is essential for identifying the root cause of performance problems.
We’re currently tuning up the convergence engine QA with Nokia’s IRA frimere, a crucial step in optimizing performance. This is closely related to the exciting new Citrix Intel tech, new Citrix Intel tech cuts virtual umbilical cord , which promises to streamline virtual connections. Ultimately, these advancements will improve the efficiency of our entire system, and we’re confident that the work on the convergence engine QA with Nokia’s IRA frimere will be significantly enhanced by these improvements.
- Performance Monitoring with IRA Frimere: IRA Frimere offers real-time performance monitoring tools. These tools provide detailed metrics on various aspects of the convergence engine, enabling users to identify performance bottlenecks quickly.
- Logging Analysis: Detailed logs from both the convergence engine and IRA Frimere are vital for understanding the sequence of events leading to performance issues. These logs provide context and often pinpoint the exact cause of degradation.
- Configuration Validation: Verifying the configuration settings within the convergence engine and IRA Frimere is critical. Misconfigurations can lead to unexpected behavior and performance problems. Ensure all parameters and dependencies are correctly set and consistent with the expected operation.
- System Resource Analysis: IRA Frimere can be used to analyze system resource usage (CPU, memory, disk I/O) during peak loads or performance degradation events. Identifying resource bottlenecks helps isolate potential causes and implement solutions.
Pinpointing Root Cause of Performance Degradation
To pinpoint the root cause, a systematic approach is necessary. Methods involve:
- Reproducing the Issue: Precisely recreating the scenario that leads to performance degradation is essential. This allows for targeted analysis of the convergence engine and IRA Frimere behavior.
- Isolate Components: Isolating components, such as specific modules within the convergence engine or IRA Frimere, allows for focusing on the problematic area.
- Comparison of Metrics: Comparing performance metrics (throughput, latency, resource usage) under different conditions helps identify the correlation between changes in conditions and performance degradation.
- Correlation Analysis: Analyzing the correlation between various factors (configuration settings, data volume, system load) can identify triggers for performance degradation.
Step-by-Step Procedure for Isolating Convergence Engine QA Problems
A structured approach is critical for effective troubleshooting.
- Reproduce the issue under controlled conditions. This involves creating a test environment that mirrors the production environment as closely as possible.
- Collect relevant logs from the convergence engine and IRA Frimere. This includes performance metrics and detailed activity logs.
- Analyze IRA Frimere logs to identify potential configuration issues or errors. Focus on sections related to convergence engine interactions.
- Identify potential bottlenecks in resource utilization. Examine CPU, memory, and disk I/O usage through IRA Frimere monitoring tools.
- Isolate the problematic component by temporarily disabling or removing parts of the system. This allows for identifying which component is causing the issue.
- Compare the performance metrics with and without the suspected component. This provides crucial insights into the component’s contribution to performance.
- Review configuration settings within the convergence engine and IRA Frimere. Ensure all parameters are correctly configured and optimized.
- Implement the necessary corrective actions. Once the root cause is identified, implement the appropriate fixes.
Using Logs and Debugging Tools in IRA Frimere
Effective log analysis is essential for understanding the behavior of the convergence engine and IRA Frimere. IRA Frimere offers specific debugging tools.
- Detailed Logging Configuration: Configure logging levels within the convergence engine and IRA Frimere to collect specific information related to performance issues.
- Filter and Search Logs: Use filtering and searching tools within IRA Frimere to quickly identify relevant logs associated with specific events or performance bottlenecks.
- Correlation of Events: Correlating events within the logs can pinpoint the precise sequence of events leading to performance issues.
- Debugging Tools within IRA Frimere: IRA Frimere provides specialized debugging tools to trace specific calls and interactions within the convergence engine.
Best Practices for Convergence Engine Tuning: Tuning Up The Convergence Engine Qa With Nokias Ira Frimere
Fine-tuning the Nokia IRA Frimere Convergence Engine for optimal performance requires a systematic approach. This involves understanding the intricacies of the engine’s architecture, identifying key performance metrics, and employing effective strategies for iterative improvement. A robust methodology, combined with clear communication and collaboration across teams, is crucial for success.
Iterative Testing and Refinement
A critical element in tuning the Convergence Engine is the iterative testing and refinement process. This involves a cyclical approach where each iteration builds upon the previous one. This cycle begins with a baseline performance evaluation, followed by targeted adjustments to specific parameters. The impact of these adjustments is then rigorously tested and measured, leading to further refinements.
Continuous monitoring and analysis of performance metrics throughout this process are vital for identifying areas for optimization and ensuring progress.
Establishing Benchmarks for Convergence Engine Performance
Establishing clear benchmarks is essential for quantifying the effectiveness of tuning efforts. These benchmarks should be measurable, specific, achievable, relevant, and time-bound (SMART). For instance, a benchmark could be to reduce the average latency for a specific type of query by 15% within a set timeframe. Benchmarking provides a quantifiable target for tuning, enabling teams to track progress and measure the impact of their efforts.
Different types of tests, from basic to complex simulations, are essential to capture various aspects of engine performance.
Communication and Collaboration Between Teams
Effective communication and collaboration are crucial throughout the tuning process. Teams responsible for the Convergence Engine, network infrastructure, application development, and QA must collaborate to identify bottlenecks and implement solutions. Regular meetings, shared dashboards, and a common understanding of the tuning goals are vital for success. Clear documentation of tuning procedures and results is essential for knowledge sharing and future optimization.
Comparison of Convergence Engine Tuning Approaches
Tuning Approach | Description | Advantages | Disadvantages |
---|---|---|---|
Manual Tuning | Adjusting parameters manually based on observation and experience. | Relatively inexpensive, allows for quick adjustments. | Prone to errors, less efficient for complex systems, and potentially inconsistent results. |
Automated Tuning | Utilizing scripts and algorithms to automate the process. | Faster, more consistent, reduces manual errors, particularly useful for large-scale tuning. | Can be more complex to implement, may require specialized expertise, might not capture nuances in specific scenarios. |
Hybrid Tuning | Combining manual and automated approaches, leveraging the strengths of both. | Balances efficiency and flexibility, allowing for focused manual adjustments where needed. | Requires careful planning and coordination to ensure smooth integration of both methods. |
This table illustrates the comparative advantages and disadvantages of each approach. Choosing the optimal method depends on factors such as the complexity of the system, available resources, and desired level of control. A hybrid approach is often the most practical, using automated tuning for repetitive tasks while allowing for manual intervention in specific problem areas.
Future Trends and Innovations
The convergence engine landscape is constantly evolving, driven by advancements in networking, cloud computing, and artificial intelligence. These shifts necessitate a forward-thinking approach to tuning and QA processes. Understanding emerging trends is crucial for ensuring the convergence engine remains adaptable and effective in the face of technological evolution.
Potential Future Trends in Convergence Engine Technology
The convergence engine of tomorrow will likely integrate more advanced features and functionalities. These trends include a move towards software-defined networking (SDN) and network function virtualization (NFV) for greater flexibility and scalability. Furthermore, increased automation and intelligent self-optimization are anticipated, leveraging machine learning and AI.
Impact on Convergence Engine Tuning Process
The integration of SDN and NFV will introduce new complexities to the tuning process. The dynamic nature of these technologies will demand adaptive tuning strategies that can react to real-time network conditions and traffic patterns. Automating tasks like configuration validation and performance monitoring becomes increasingly important.
Nokia IRA Frimere’s Evolution
Nokia’s IRA Frimere will need to adapt to accommodate these technological advancements. This includes developing tools and frameworks that support the automation of tuning tasks and integrate with SDN and NFV environments. Enhanced integration with AI-driven optimization platforms is another crucial direction for future development.
Automation in Convergence Engine QA and Tuning
Automation promises to significantly streamline the QA and tuning process. Automated testing tools and scripts can reduce manual effort, improve consistency, and accelerate the overall process. Automated performance monitoring tools can also proactively identify and address potential bottlenecks, reducing downtime and enhancing stability.
Role of AI in Improving Convergence Engine Performance
AI can play a crucial role in optimizing convergence engine performance. AI algorithms can analyze vast amounts of network data to identify patterns, predict future performance issues, and suggest optimal configurations. This proactive approach can minimize the risk of performance degradation and improve overall system reliability. Machine learning models, trained on historical data, can provide insights into how the network will react to different traffic loads and conditions.
Final Thoughts
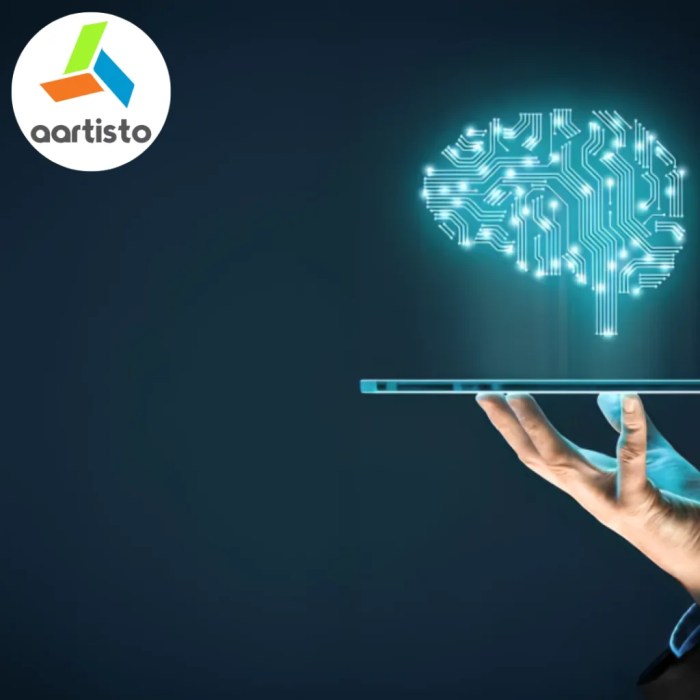
In conclusion, tuning up the convergence engine QA with Nokia’s IRA Frimere involves a multifaceted approach. From understanding the engine’s core functions and performance metrics to utilizing IRA Frimere’s capabilities, this process necessitates careful consideration of various factors. By adhering to best practices and proactively addressing potential issues, we can ensure optimal performance and a superior user experience.
The future of convergence engine technology promises even greater complexity, requiring continuous adaptation and innovation in tuning strategies.