Will Microsofts Kumo Revolutionize Search?
Will microsofts kumo bring new visual dimension to search – Will Microsoft’s Kumo bring new visual dimension to search? This exploration dives into the potential of Kumo, Microsoft’s innovative technology, to transform how we interact with search engines. Current search methods often rely heavily on text-based queries, but Kumo promises a more intuitive and visually-rich experience. We’ll examine Kumo’s visual recognition capabilities, potential impact on user experience, and the technical aspects behind this exciting advancement.
Imagine searching for a specific type of antique furniture. Instead of typing s, you upload a picture of the item. Kumo analyzes the image, identifying key features and returning results tailored to the visual characteristics. This visual approach promises greater accuracy and relevance compared to traditional text-based searches. We’ll also compare Kumo to other visual search technologies to understand its potential position in the market.
Introduction to Microsoft’s Kumo and Search: Will Microsofts Kumo Bring New Visual Dimension To Search
Microsoft’s Kumo is a new search technology that promises a revolutionary approach to information retrieval. It leverages cutting-edge AI and machine learning to provide more intuitive and comprehensive search results. While the exact implementation details are still emerging, early reports suggest a focus on visual data, making it a potential game-changer in how we interact with and understand information.
The current state of search technology is largely text-based, relying on s and algorithms to match queries with relevant documents. However, this approach often falls short in capturing the richness and context of visual data, leading to fragmented and incomplete results. Kumo’s potential impact on search is significant, as it aims to bridge this gap by integrating visual understanding into the search process.
This could lead to more accurate and insightful search results, allowing users to find what they need more efficiently.
Overview of Microsoft’s Kumo Technology
Kumo, a likely abbreviation for “Knowledge Understanding and Modelling,” is a relatively new development in Microsoft’s AI ecosystem. It utilizes advanced computer vision techniques to analyze and interpret images, videos, and other visual content. This goes beyond simple image recognition, aiming to extract semantic meaning and contextual information from visuals. By understanding the relationships between visual elements and associating them with textual data, Kumo has the potential to provide a more holistic understanding of information.
Current State of Search Technology
Current search technology heavily relies on text-based indexing and retrieval. Search engines use algorithms to identify s in documents and match them with user queries. While effective for text-based information, this approach struggles with visual data. Users often need to resort to separate image search tools, which are less integrated with broader search results. This fragmented approach limits the depth and breadth of information users can access.
Potential Impact of Kumo on Search, Focusing on Visuals
Kumo’s integration of visual understanding into search has the potential to transform how we find and use information. Instead of just searching for images containing specific objects, users could ask more nuanced questions, such as “Find articles about buildings with similar architectural styles to this image” or “Show me videos of people using this type of tool in different contexts.” This contextual understanding allows for more comprehensive and relevant search results, especially in fields like design, history, and social sciences.
Comparison of Kumo to Other Visual Search Technologies
Feature | Kumo | Other Visual Search Technologies |
---|---|---|
Data Source | Images, videos, other visual formats, potentially integrated with text data. | Primarily images, potentially with limited support for other formats. |
Semantic Understanding | Aims for deep semantic understanding of visuals, including relationships and context. | Often limited to object recognition or basic image matching. |
Integration with Text Search | Expected to seamlessly integrate visual and textual data, creating more comprehensive results. | Usually operates as separate systems, making cross-referencing difficult. |
Scalability | Microsoft’s resources suggest potential for handling vast amounts of visual data. | Scalability varies significantly based on the specific technology. |
Visual Search Capabilities of Kumo
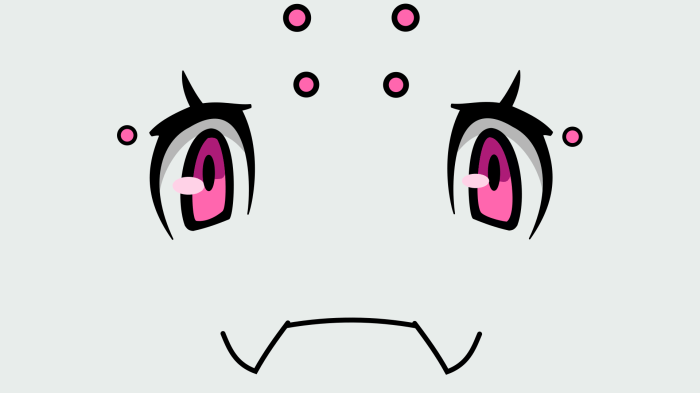
Microsoft’s Kumo, the upcoming search technology, promises a revolutionary shift in how we interact with information. Beyond simple searches, Kumo aims to unlock the power of visual data, enabling users to find what they need by simply uploading an image. This innovative approach to search holds significant potential for improved accuracy and relevance, particularly in areas like product identification, historical research, and artistic discovery.Kumo’s visual recognition capabilities are based on advanced computer vision algorithms.
These algorithms analyze the unique characteristics of images, enabling Kumo to identify objects, scenes, and even specific instances of something within a picture. This surpasses the limitations of traditional -based searches, which rely on textual descriptions and can struggle with ambiguity or nuanced details. Kumo’s processing of images involves breaking them down into their constituent parts, recognizing patterns, and then matching those patterns against a vast database of known objects and concepts.
Will Microsoft’s Kumo bring a fresh visual dimension to search? It’s a fascinating question, and perhaps the answer lies in the success of previous formats. Think about what Blu-ray won – what did Blu-ray win – in terms of visual quality and user experience. Ultimately, Kumo’s success will depend on whether it can build on those foundations and offer a genuinely superior visual search experience.
This sophisticated process allows Kumo to understand the context of the image and provide more precise results.
Visual Recognition Algorithms
Kumo employs a combination of deep learning models and image processing techniques. Deep learning models, specifically convolutional neural networks (CNNs), are trained on massive datasets of images, enabling them to learn complex visual patterns. These networks can identify objects, scenes, and even subtle details, such as textures, colors, and shapes. Image processing techniques further refine the analysis, extracting features that are relevant to the search query.
This combination of powerful algorithms allows Kumo to understand the nuances of visual data, ultimately enhancing search accuracy.
Comparison with Textual Search
Traditional textual search methods rely on s and metadata. While effective for finding information based on text, they often fall short when dealing with visual information. Kumo, in contrast, leverages the power of visual recognition to extract meaning from images, offering a more intuitive and accurate way to search for visual content. Imagine trying to find a specific type of antique furniture.
A textual search might only yield results if the furniture’s description is included in the database. Kumo, however, could identify the furniture through an image, even if its description is missing or incomplete.
Visual Search Scenarios, Will microsofts kumo bring new visual dimension to search
Scenario | Kumo’s Potential Handling |
---|---|
Identifying a specific plant in a photograph | Kumo would analyze the image, recognizing the plant’s characteristics, and comparing it against its database to pinpoint the species. |
Finding similar products based on a picture of an item | Kumo could identify the product in the image and retrieve similar products from its database, factoring in features like color, shape, and design. |
Locating a specific historical event depicted in a painting | By analyzing the image, Kumo could identify figures, objects, and locations, enabling a precise search for historical events that match the painting’s content. |
Finding similar styles of architecture based on a picture of a building | Kumo could recognize architectural features and styles, providing a list of buildings with comparable characteristics. |
Impact on User Experience
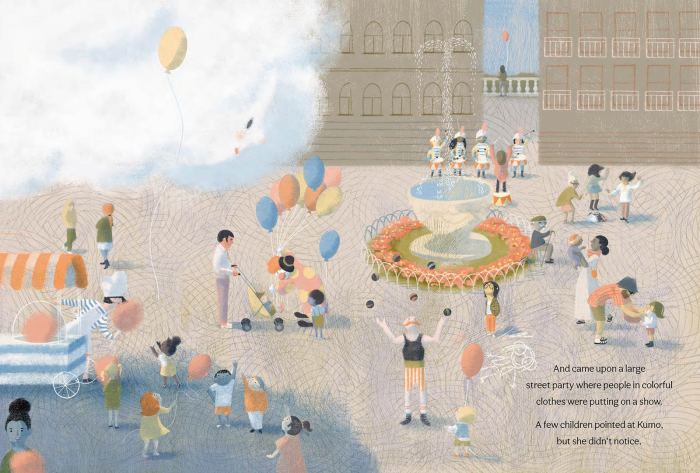
Visual search, powered by Microsoft’s Kumo, promises a significant shift in how we interact with search engines. It moves beyond -based queries, enabling users to connect with information through images, videos, and other visual representations. This approach is poised to revolutionize the user experience, making search more intuitive and fulfilling.
Potential Alterations in User Interaction
The fundamental shift in user interaction with Kumo-powered visual search stems from the ability to query by visual context rather than textual descriptions. Users will likely engage in more exploratory searches, using images as starting points for information discovery. Instead of typing a lengthy description of a product, a user could simply upload an image and find related items, similar designs, or detailed information.
This visual approach allows for a more natural and less restrictive way to formulate queries.
Microsoft’s Kumo project intrigues me – will it truly revolutionize search with new visuals? It’s fascinating to consider how search could evolve, but the question of whether or not shortcovers could potentially impact the Kindle’s future sales is also a very interesting point. Could shortcovers throw water on the Kindle? Ultimately, I’m still curious to see if Kumo’s visual approach will offer a genuinely new dimension to searching online.
Expected User Experience Improvements
Kumo’s integration into search promises a more intuitive and efficient search experience. The ability to search using visual cues will dramatically improve accuracy and relevance, providing users with more targeted and useful results. Users will experience less frustration from irrelevant results, as the search engine will understand the visual context of the query. Imagine quickly identifying a specific style of furniture by uploading a picture of a detail, rather than meticulously describing it in text.
This direct visual connection will likely reduce the cognitive load associated with traditional text-based searches.
Potential Benefits and Drawbacks for Users
The benefits of visual search are substantial. Users gain the ability to discover products, ideas, and information with unprecedented speed and accuracy. They can visually explore diverse topics and find connections between seemingly disparate concepts. However, there are potential drawbacks to consider. One concern is the potential for misinterpretation of images, leading to inaccurate results.
Another challenge is the need for users to understand how to effectively utilize visual search queries. Furthermore, the sheer volume of visual data that Kumo must process could potentially lead to slower query times if not optimized effectively.
Comparison of Current and Potential Kumo-Powered Interfaces
Feature | Current Search Engine Interface | Potential Kumo-Powered Interface |
---|---|---|
Query Method | Text-based s, advanced operators | Image uploads, visual object recognition, contextual searches |
Result Presentation | List of web pages, snippets, and links | Visual representations of results, augmented reality previews (if applicable), interactive product visualizations |
User Interaction | Typing, refining queries, using filters | Uploading images, adjusting visual parameters, exploring related visuals |
Accuracy | Dependent on accuracy and user understanding of search operators | Potentially higher accuracy through visual recognition and contextual understanding |
Time to Find Results | Variable, often dependent on search query clarity | Potentially faster results through visual queries |
The table highlights the fundamental difference in how users will interact with Kumo-powered search. It illustrates the shift from a textual search paradigm to a visual one, emphasizing the potential for a more intuitive and efficient user experience.
Technical Aspects and Implementation
Microsoft’s Kumo visual search, a key component of its enhanced search capabilities, relies on a sophisticated technical architecture. This architecture integrates advanced image processing, powerful machine learning models, and a robust data infrastructure to deliver accurate and intuitive visual search results. The implementation of Kumo involves complex but crucial steps, each designed to improve the user experience and ensure scalability.The technical underpinnings of Kumo’s visual search are multifaceted.
Will Microsoft’s Kumo truly revolutionize search with a fresh visual approach? It’s a fascinating question, especially considering Microsoft’s broader initiative to boost American tech literacy, like their program to microsoft aims to bring americans up to tech speed. Ultimately, Kumo’s success in delivering a truly innovative visual search experience will depend on how well it bridges the gap between user expectations and technical implementation.
The potential for a new visual dimension in search is certainly exciting.
It’s not simply a matter of pointing a camera at something and getting results; instead, it’s a sophisticated process of analyzing visual data and matching it to relevant information within its vast knowledge base. This requires advanced algorithms and robust infrastructure.
Data Processing Pipeline
The process of turning an image into a usable search query involves a multi-stage pipeline. First, the image is preprocessed, adjusting for lighting, orientation, and other variations to ensure consistency in the analysis. Then, the image undergoes feature extraction, identifying key visual elements like shapes, colors, and textures. These extracted features are crucial for comparison and matching against existing data.
The pipeline then uses machine learning models to analyze the extracted features and identify the most probable matches within the dataset.
Machine Learning Techniques
Kumo leverages various machine learning techniques to enhance visual recognition. Deep learning models, particularly convolutional neural networks (CNNs), are a cornerstone of this process. These models learn complex patterns and relationships within image data, enabling accurate identification of objects and scenes. Transfer learning, a technique where models trained on a large dataset are adapted for a specific task, plays a crucial role in improving efficiency and accuracy.
This reduces the need for extensive training data specific to Kumo’s use case. For example, a model pre-trained on a vast image dataset could be fine-tuned for identifying specific product categories.
Scalability and Performance
Integrating visual search into a platform like Microsoft Search necessitates a highly scalable architecture. The system must handle a massive volume of images and queries efficiently. This involves distributing the workload across multiple servers, optimizing data storage, and utilizing caching mechanisms to reduce latency. For instance, Kumo might utilize cloud-based infrastructure to dynamically adjust resources based on demand.
This ensures that the system remains responsive even during periods of high traffic. The performance implications are crucial, impacting user experience directly. Slow responses lead to frustration, highlighting the importance of optimized query processing and data access.
Technical Requirements for Implementation
Implementing Kumo’s visual search capabilities requires a robust set of technical specifications. These specifications are vital to ensure the system’s accuracy, reliability, and scalability.
Category | Requirement |
---|---|
Data Storage | High-capacity storage solution capable of handling massive image datasets, optimized for fast retrieval and indexing. |
Processing Power | High-performance computing resources to process image data, extract features, and run machine learning models efficiently. |
Network Infrastructure | High-bandwidth network to facilitate the transfer of image data and query results between different components of the system. |
Machine Learning Models | Pre-trained and fine-tuned deep learning models, including CNNs, for accurate image recognition and object detection. |
Security | Robust security measures to protect sensitive data and ensure the confidentiality and integrity of user queries. |
Scalability | Scalable architecture capable of handling a large volume of concurrent user requests and expanding to accommodate future growth. |
Future Implications and Potential Applications
Microsoft’s Kumo, with its visual search capabilities, holds immense potential to reshape numerous industries. Its ability to understand and interpret visual data promises a paradigm shift in how we interact with information and access knowledge. This opens doors to new applications and workflows, transforming the way we learn, shop, work, and engage with the world around us.
Potential to Revolutionize Industries
Kumo’s visual search technology can revolutionize various industries by enabling a more intuitive and efficient approach to information retrieval. Imagine identifying a specific product in a crowded store simply by snapping a picture or finding similar items based on a visual cue. This efficiency is not limited to retail; it extends to fields like healthcare, education, and even the arts.
Examples of Visual Search Applications
Visual search can be applied across a broad spectrum of contexts. In the retail sector, customers could easily identify and purchase products they see in a magazine or online advertisement by taking a picture of the item. In the medical field, Kumo could assist in diagnosing illnesses by comparing visual patterns in medical images with a vast database of similar cases.
Imagine a chef finding similar recipes by snapping a picture of an unfamiliar dish. Education can benefit from visual search in locating and understanding complex images or diagrams in textbooks. Even the arts can be enriched, with artists and researchers identifying similar styles or motifs in paintings or sculptures.
Potential Limitations and Challenges
Despite its immense potential, Kumo’s visual search technology faces certain limitations. Accuracy in image recognition is crucial, but challenges exist in distinguishing subtle variations or complex patterns. The sheer volume of visual data that needs to be processed and indexed poses a significant computational challenge. Data privacy and security concerns also arise when dealing with sensitive visual information.
Moreover, the need for extensive training data and algorithms to recognize diverse objects and contexts across different cultures and languages is crucial.
Table of Potential Applications
Industry | Potential Application | Emerging Field Implications |
---|---|---|
Retail | Product identification, personalized recommendations, virtual try-ons | Enhanced shopping experience, reduced product return rates |
Healthcare | Medical image analysis, disease diagnosis, drug discovery | Improved diagnostic accuracy, faster treatment development |
Education | Visual learning resources, historical artifact identification, language learning | Enhanced student engagement, accessibility to global knowledge |
Arts & Culture | Art style identification, historical analysis, virtual museum experiences | Preservation of cultural heritage, deeper engagement with art |
Manufacturing | Quality control, defect detection, maintenance scheduling | Increased efficiency, reduced downtime, improved product quality |
Agriculture | Crop identification, pest detection, yield prediction | Precision agriculture, enhanced food security |
Illustrative Examples of Visual Search
Microsoft’s Kumo search, with its visual capabilities, promises a revolution in how we interact with information. Instead of relying solely on s, Kumo allows users to search using images, unlocking a whole new dimension of discovery. This shift opens up exciting possibilities for various fields, from academic research to everyday tasks.
Scenarios for Visual Search
Visual search is particularly useful in situations where traditional text-based searches fall short. Imagine trying to find a specific type of antique furniture, or identifying a rare plant in a botanical garden. Traditional search engines might struggle with such tasks, while Kumo’s visual capabilities excel.
Examples of Visual Search in Action
- Identifying Products: A user might upload an image of a specific shoe they saw in a store but couldn’t remember the brand. Kumo could then identify the shoe model and display results for purchasing options, providing detailed information on the product, including its features, price, and availability. This is more efficient than searching through pages of footwear descriptions.
- Finding Similar Items: A user could upload a picture of a painting they admire. Kumo could locate similar works of art, providing details on artists, styles, and even historical context. This allows users to discover new pieces based on their existing preferences.
- Locating Landmarks: Users can capture a photo of a building or monument and use Kumo to locate its precise address, learn its history, or find nearby attractions. This makes navigating unfamiliar cities more intuitive and efficient.
- Identifying Plants: Users can take a picture of an unknown plant and Kumo can identify the species. This could be valuable for gardeners, botanists, or anyone interested in plant identification.
Visual Representation of Search Results
Kumo’s search results are presented in a visually engaging format. Instead of just text links, the results would likely incorporate images of matching items or similar objects. A user searching for a specific type of chair, for instance, would see a gallery of chairs with detailed information presented alongside each image. This format facilitates quick comparisons and enhances user comprehension.
Comparison of Visual vs. Text-Based Search Results
Search Query | Visual Search Results | Text-Based Search Results |
---|---|---|
Image of a vintage car | Gallery of similar vintage cars, details on models, historical information, and links to related articles. | Web pages containing text about vintage cars, potentially links to images, but not a visually-driven presentation of similar cars. |
Picture of a flower | Identification of the flower species, information on its characteristics, care instructions, and related plant varieties. | Web pages containing text about flowers, potentially links to images, but lacking the specificity of identifying the exact species. |
Screenshot of a logo | Results showing similar logos, details about the company, and possibly historical information on the brand. | Web pages containing text about the company, potentially links to the company’s website. |
Final Wrap-Up
Ultimately, will Microsoft’s Kumo redefine search? The potential is enormous, promising a more intuitive and visually driven search experience. While technical hurdles and user adoption remain to be seen, Kumo’s capabilities, if fully realized, could dramatically shift how we discover information. The future of search may be more visual than ever before, and Kumo could be the key to unlocking this new dimension.