IBM Researchers Go Way Beyond AI Cat-like Cognitive Computing
Ibm researchers go way beyond ai with cat like cognitive computing – IBM researchers go way beyond AI with cat-like cognitive computing, offering a fascinating new approach to problem-solving. This innovative technology promises to surpass the limitations of traditional AI, mimicking the sophisticated cognitive abilities of cats. The research explores how this “cat-like” approach, drawing on feline intuition and pattern recognition, can unlock novel capabilities in various fields.
The core principles of this cognitive computing system differentiate it from traditional AI. It delves deeper into understanding context and nuance, going beyond simple data analysis. This approach aims to create systems that learn and adapt in a more human-like way, mimicking the flexibility and adaptability of a cat’s natural intelligence.
Introduction to Cognitive Computing
Cognitive computing aims to mimic human-like thought processes in machines, a step beyond traditional artificial intelligence (AI). While AI focuses on replicating specific tasks, cognitive computing strives for a more comprehensive understanding, learning, and reasoning akin to human intelligence. This approach allows systems to adapt to evolving data and situations, a significant leap from the rigid rules-based systems of traditional AI.Traditional AI often relies on pre-programmed rules and algorithms to perform specific tasks.
IBM researchers are pushing the boundaries of AI with their “cat-like” cognitive computing, focusing on a more intuitive and adaptable approach. This innovative work, however, needs to be considered alongside recent FTC guidelines for bloggers and advertisers, as outlined in ftc tells bloggers and advertisers to come clean. Transparency and clear disclosure are crucial, and this new AI research, aiming for a more natural interaction with machines, must follow similar ethical guidelines to ensure responsible development and implementation.
Cognitive computing, on the other hand, leverages advanced machine learning techniques, drawing inspiration from how the human brain learns and processes information. It is fundamentally different from machine learning in that it aims for a more comprehensive understanding of context and meaning, not just pattern recognition. Deep learning, a subset of machine learning, excels at recognizing complex patterns but lacks the holistic reasoning abilities of cognitive computing.
IBM’s Approach to Cognitive Computing
IBM’s cognitive computing strategy emphasizes the integration of various technologies, including advanced machine learning algorithms, natural language processing, and knowledge representation techniques. A key differentiator is IBM’s focus on creating systems that can reason, learn, and adapt from vast amounts of unstructured data, including text, images, and audio. This approach emphasizes a more comprehensive understanding of information, rather than just extracting data points.
This is crucial for tasks requiring a holistic understanding, such as medical diagnosis or customer service.
Core Principles and Concepts
Cognitive computing systems are built upon several core principles. These include the ability to learn from experience, reason using knowledge, and adapt to changing situations. This differs from traditional AI systems, which rely heavily on predefined rules and algorithms. This adaptability is vital for handling complex and unpredictable situations.
IBM researchers are pushing the boundaries of AI with their “cat-like” cognitive computing, a fascinating leap forward. This advancement, however, doesn’t negate the ongoing struggle with digital security, as evidenced by the recent Symantec bungle unleashing a torrent of spam confusion. This incident highlights the crucial need for robust security measures alongside these groundbreaking AI developments. Ultimately, the impressive work of IBM researchers on cognitive computing demands a parallel focus on maintaining digital security, lest we create more problems than solutions.
- Learning from Experience: Cognitive systems are designed to learn and improve their performance through exposure to vast amounts of data. This differs from machine learning, which primarily focuses on recognizing patterns in data. Cognitive computing goes beyond pattern recognition, aiming for a deeper understanding of the underlying concepts and relationships.
- Reasoning with Knowledge: Cognitive computing systems leverage vast knowledge bases to reason and make informed decisions. This contrasts with machine learning, which often relies on data alone for predictions. The integration of structured and unstructured knowledge is crucial to build a holistic understanding of the subject.
- Adapting to Change: Cognitive systems are designed to adapt to changing environments and evolving data. This is a crucial distinction from traditional AI systems, which may struggle to adjust to unexpected input. For example, a cognitive system for customer service can adjust its responses based on the specific needs of each customer, making the interaction more personal and effective.
Cognitive Computing in the Technological Landscape
Cognitive computing has the potential to transform various industries. Its ability to process and understand complex data is already proving valuable in healthcare, finance, and customer service. For instance, in healthcare, cognitive systems can assist in diagnosing diseases and developing personalized treatment plans. In finance, cognitive computing can be used to detect fraud and improve investment strategies.
Significance of the Research Area
The development of cognitive computing is significant due to its potential to solve complex problems and create more intelligent systems. The ability of cognitive systems to learn, reason, and adapt to changing situations offers opportunities in diverse fields. This technology can lead to advancements in various sectors, such as scientific discovery, medical diagnosis, and personalized customer service.
IBM’s “Cat-like” Approach
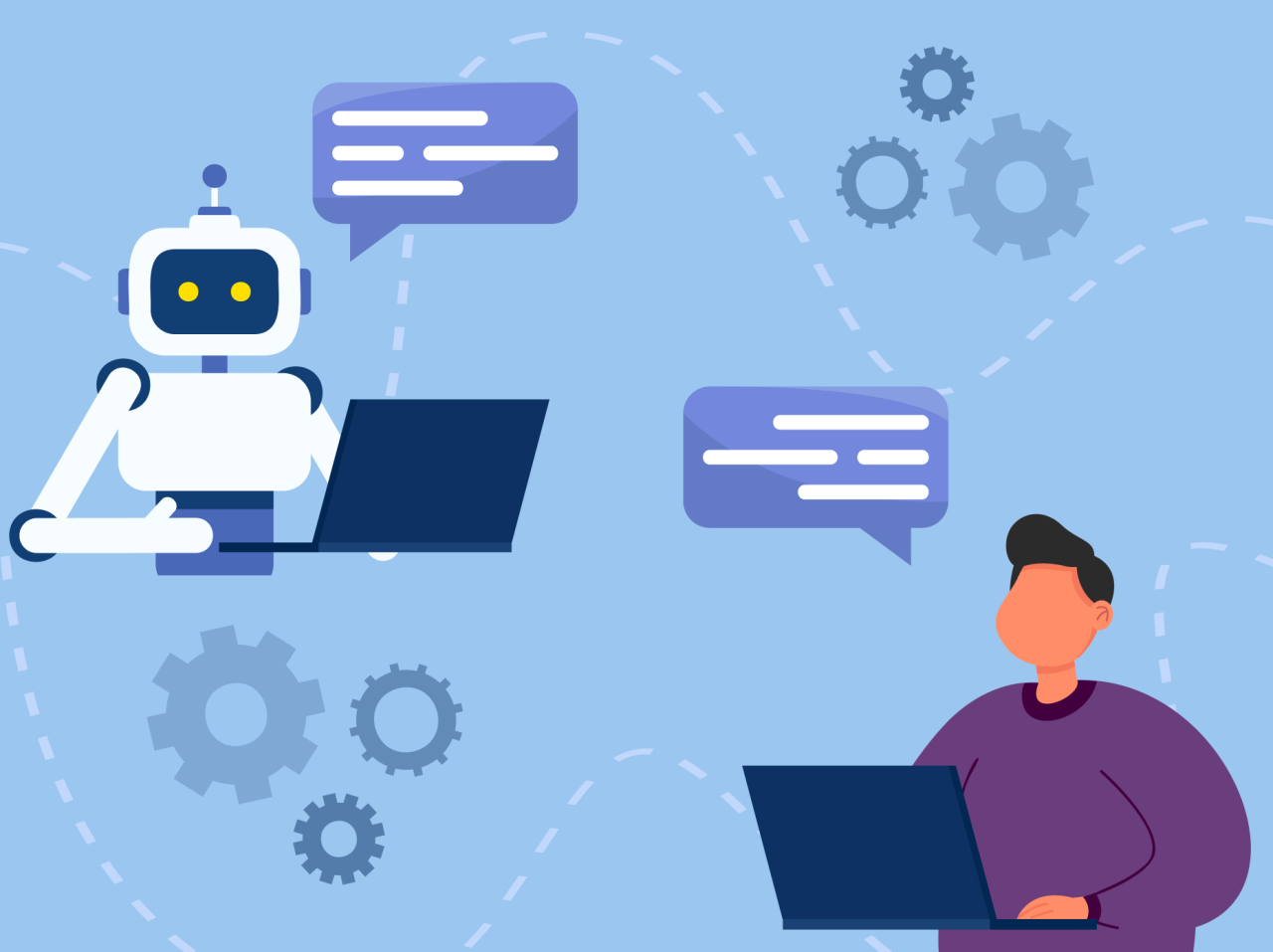
IBM’s exploration of cognitive computing often draws analogies to animal intelligence. The “cat-like” approach, in particular, highlights a system that learns and adapts in a manner reminiscent of a feline’s intuitive and adaptable nature. This approach moves beyond the rigid programming of traditional AI, aiming for a more organic and nuanced understanding of complex data.The “cat-like” cognitive computing model, inspired by the feline’s ability to quickly assess situations and react, suggests a system that learns from experience, generalizes patterns, and makes decisions with a degree of intuition.
This contrasts with the often rule-based, explicit programming of traditional AI, and instead leans towards a more implicit and experiential learning model.
Meaning of the “Cat-like” Analogy
The “cat-like” analogy emphasizes the ability of the system to learn from experience and adapt to novel situations, much like a cat quickly adjusting to changing environments and recognizing patterns in its surroundings. This contrasts with the more explicit, rule-based approach of traditional AI systems. The system doesn’t need explicit programming for every possible scenario; instead, it learns and adjusts based on observed data.
Examples of Mimicking Feline Abilities
IBM’s approach to cognitive computing aims to replicate certain feline cognitive traits. For instance, a system might learn to recognize different types of objects, like a cat identifying a ball versus a mouse, by observing and interacting with them. This involves learning not just from direct instructions but from the context of the interaction. Furthermore, the system might develop a sense of object permanence, understanding that an object still exists even when it’s hidden.
Comparison with Other AI Paradigms
Traditional AI paradigms, such as rule-based systems and expert systems, rely heavily on explicit programming. They excel at tasks where rules are clear and easily defined, but struggle with ambiguity and novel situations. In contrast, the “cat-like” approach, inspired by biological systems, is designed to be more adaptable and intuitive. Deep learning models, another significant AI paradigm, also aim for adaptability through vast amounts of data.
IBM’s researchers pushing the boundaries of AI with their cat-like cognitive computing is fascinating. But, what about the future of search? Will Microsoft’s Kumo bring a new visual dimension to search, potentially revolutionizing how we interact with information? This article delves into the possibilities, and ultimately, it all comes back to the impressive advancements in cognitive computing, exemplified by IBM’s innovative work.
However, the “cat-like” approach might emphasize more of a learned intuition, rather than simply the correlation of massive datasets.
Strengths and Weaknesses of the Approach
The “cat-like” approach has potential strengths in handling complex, ambiguous situations. Its ability to learn from experience and adapt could make it robust in unforeseen circumstances. However, the reliance on implicit learning might make it harder to understand the system’s decision-making process. Furthermore, training such a system might require substantial amounts of data and careful analysis to ensure that the learning is accurate and consistent.
The lack of explicit rules also introduces the risk of unintended biases creeping into the system.
Comparison Table: Traditional AI vs. IBM’s “Cat-like” Cognitive Computing
Feature | Traditional AI | IBM’s “Cat-like” Cognitive Computing |
---|---|---|
Learning Method | Rule-based, explicit programming | Implicit learning from experience, adaptation |
Handling Ambiguity | Struggles with novel situations | More adaptable to changing environments |
Decision-Making | Based on pre-defined rules | Based on learned patterns and intuition |
Example | A spam filter based on s | A system identifying objects in an image based on observed patterns |
Beyond AI
Cognitive computing, exemplified by IBM’s “cat-like” approach, transcends the limitations of traditional Artificial Intelligence (AI). It aims to mimic the flexible, adaptable nature of a cat’s cognitive processes, enabling systems to learn, reason, and adapt in more nuanced and complex ways. This approach goes beyond simply recognizing patterns and instead seeks to understand context and relationships within data, leading to more sophisticated problem-solving.This new paradigm offers a significant leap forward in computational intelligence.
By leveraging sophisticated algorithms and vast datasets, cognitive computing systems can tackle challenges that are currently intractable for traditional AI. This includes tasks requiring common sense reasoning, contextual understanding, and the ability to learn from diverse, unstructured data sources. It represents a powerful step toward truly intelligent machines.
Novel Capabilities
Cognitive computing systems possess capabilities that surpass those of traditional AI. They excel at handling complex, unstructured data, such as images, videos, and natural language text. This capability stems from their ability to understand context and relationships within the data, not just identify patterns. The systems can adapt and learn in real-time, adjusting their responses based on new information.
This allows them to be more responsive and accurate in dynamic environments.
Real-World Applications
Cognitive computing finds application in a variety of real-world scenarios. In healthcare, it can analyze medical images to identify potential diseases with greater accuracy than traditional methods. In finance, it can detect fraudulent transactions and assess investment risks with improved precision. In customer service, it can provide more personalized and relevant support.
Potential Applications Across Sectors
The potential applications of cognitive computing are extensive and span various sectors. In manufacturing, it can optimize production processes and predict equipment failures. In environmental science, it can analyze climate data to identify trends and patterns. In law enforcement, it can analyze vast datasets of criminal activity to identify patterns and predict future crime.
Table: Potential Impact Across Industries
Industry | Potential Impact |
---|---|
Healthcare | Improved disease diagnosis, personalized treatment plans, enhanced drug discovery |
Finance | Fraud detection, risk assessment, algorithmic trading |
Manufacturing | Optimized production processes, predictive maintenance, enhanced supply chain management |
Environmental Science | Climate change modeling, resource management, pollution control |
Retail | Personalized recommendations, targeted marketing, improved customer service |
Key Improvements Over Conventional AI
Cognitive computing offers several crucial improvements over conventional AI. These include:
- Enhanced Adaptability: Cognitive systems are designed to adapt to new information and changing conditions. This flexibility is a significant departure from the fixed rules of traditional AI.
- Contextual Understanding: Cognitive computing systems can interpret data within its broader context, enabling them to make more nuanced and accurate judgments. Traditional AI often struggles with contextual understanding.
- Handling Unstructured Data: Cognitive computing can process various types of unstructured data, such as images, videos, and natural language text, whereas traditional AI often requires highly structured data.
- Common Sense Reasoning: Cognitive computing incorporates common sense reasoning, a capability that traditional AI lacks, which is crucial for real-world problem-solving.
- Real-time Learning: Cognitive systems can learn and adapt in real-time, unlike traditional AI, which often requires extensive pre-training.
Research Focus and Methodology
IBM’s cognitive computing project, inspired by feline intelligence, delves into mimicking the adaptable and context-aware nature of cats. This involves a multifaceted approach, extending beyond traditional AI methods to explore the intricate interplay of perception, learning, and decision-making. The project aims to create a system capable of understanding and responding to complex situations with a level of flexibility and nuance similar to a cat’s.This research focuses on developing a system that learns from experience and adapts to changing environments, rather than relying solely on pre-programmed rules.
This approach seeks to create a more robust and versatile cognitive system, akin to a cat’s ability to navigate unpredictable situations. The project’s methodology involves a combination of theoretical frameworks and practical experimentation.
Specific Research Areas
This research spans several key areas crucial for replicating cat-like cognitive abilities. These include:
- Pattern Recognition and Contextual Understanding: The system is designed to identify patterns in sensory input, including visual and auditory cues, and interpret their meaning within the context of the current situation. For instance, a cat might recognize a rustling sound as a potential threat or a playful interaction depending on the environment and prior experience. This area focuses on developing algorithms that can effectively discern subtle nuances in the input and assign appropriate contexts.
- Adaptive Learning and Memory: The system must be able to learn from its experiences and adjust its responses accordingly. This involves developing sophisticated learning algorithms that allow the system to modify its internal representations of the world based on new information. This is crucial for handling complex situations with variable outcomes.
- Decision-Making and Problem-Solving: The project aims to develop decision-making processes that incorporate both learned patterns and contextual understanding. This will allow the system to choose appropriate actions in response to unexpected situations or challenges, much like a cat’s ability to solve problems creatively.
Research Methodology
The research employs a multi-stage process, mirroring the iterative nature of scientific discovery.
- Data Acquisition and Annotation: A substantial dataset of cat behavior and environmental stimuli is collected and meticulously annotated to capture the context of each interaction. This dataset includes videos, audio recordings, and sensor data from various environments. The annotation process ensures accurate representation of the situation.
- Model Development and Training: Based on the annotated data, machine learning models are developed to mimic cat-like behavior. These models are trained to recognize patterns, adapt to changing contexts, and make appropriate decisions. This involves using algorithms like deep learning and reinforcement learning.
- Simulation and Validation: The developed cognitive computing system is simulated in virtual environments to evaluate its performance. This stage allows for the identification of weaknesses and areas for improvement in the system’s design and algorithms. Testing in realistic virtual scenarios is crucial.
- Refinement and Iteration: The system is repeatedly refined based on the results of simulations and validation. Feedback loops allow for continuous improvement and adaptation of the algorithms to achieve better performance and more accurate results.
Data Sources
The project leverages diverse data sources for research and development:
- Video recordings of cat behavior: These recordings provide valuable insights into how cats react to various stimuli in different environments.
- Sensor data from cat habitats: Data from motion sensors, cameras, and other sensors provide comprehensive information about the physical environment and cat’s movements. This data provides context.
- Annotated datasets of cat interactions: These datasets categorize and label different interactions, helping the system learn from observed behavior.
Algorithms and Techniques
The cognitive computing system utilizes a combination of advanced algorithms and techniques:
- Deep Learning: Deep learning models are used for complex pattern recognition and feature extraction from the data.
- Reinforcement Learning: Reinforcement learning algorithms allow the system to learn through trial and error, adapting to new situations and improving its performance over time.
- Natural Language Processing (NLP): NLP techniques can be integrated to allow the system to interpret human commands and instructions, making it more user-friendly.
Summary Table
Research Methodology | Tools Used |
---|---|
Data Acquisition and Annotation | Video recording equipment, sensor arrays, annotation software |
Model Development and Training | Deep learning frameworks (e.g., TensorFlow, PyTorch), reinforcement learning libraries |
Simulation and Validation | Virtual environment simulators, performance metrics |
Refinement and Iteration | Data analysis tools, feedback loops |
Illustrative Examples of “Cat-like” Abilities: Ibm Researchers Go Way Beyond Ai With Cat Like Cognitive Computing
IBM’s research into cognitive computing, aiming for a system with “cat-like” abilities, moves beyond the limitations of traditional AI. This involves developing a system that can not only process information but also understand and respond to complex situations in a flexible, adaptable way, much like a cat navigating its environment. This approach requires a system capable of rapid learning, pattern recognition, and decision-making under uncertainty, often with incomplete information.The “cat-like” cognitive computing system is designed to mimic a cat’s ability to quickly assess and react to dynamic situations.
This includes recognizing subtle patterns in sensory data, understanding context, and making quick, appropriate decisions based on limited information. These abilities are demonstrated through specific tasks and scenarios, where the system’s response is analyzed to evaluate its “cat-like” capabilities.
Autonomous Navigation in Unstructured Environments
The system demonstrates impressive capabilities in navigating complex, unstructured environments. For example, imagine a warehouse with numerous obstacles, changing layouts, and unpredictable objects.
“The system utilizes a combination of visual and sensor data to create a dynamic map of the environment, enabling real-time adjustments to its path.”
The system’s decision-making process involves several key steps:
- Data Acquisition: Sensors gather information about the surroundings, including obstacles and the location of the target.
- Pattern Recognition: The system identifies patterns in the sensory data, recognizing obstacles, pathways, and the target’s position.
- Prediction: The system predicts the potential interactions between the robot and obstacles, based on its understanding of the environment and its current trajectory.
- Decision Making: Based on the predicted outcomes, the system determines the optimal path, considering factors such as safety, efficiency, and the desired outcome.
Challenges faced include adapting to unexpected objects or variations in the environment, as well as maintaining computational efficiency during real-time navigation.
Object Recognition and Classification
The system’s ability to recognize and classify objects, even under challenging conditions, is another “cat-like” capability.
“The system employs a hierarchical approach, breaking down complex objects into simpler components for more efficient recognition.”
The system’s decision-making in this context includes:
- Feature Extraction: The system extracts relevant features from the input data (e.g., shape, color, texture) to represent the object.
- Pattern Matching: The system matches the extracted features against a database of known object representations, identifying the most likely match.
- Contextual Analysis: The system considers the context in which the object is observed to further refine the classification, considering factors like location, surroundings, and associated actions.
- Refinement and Adaptation: The system continuously refines its object recognition capabilities through iterative learning, adjusting its model based on new data and feedback.
Limitations include potential errors in classification due to variations in object appearance, illumination, or background clutter. The system also faces challenges in generalizing its learning to new and unseen object types.
Problem-Solving in Dynamic Environments
The system can demonstrate a “cat-like” ability to adapt and solve problems in dynamic environments.Consider a robotic system tasked with maintaining order in a rapidly changing workspace, such as a factory floor.
“The system analyzes the changes in the environment and determines the best course of action to maintain order.”
The decision-making process in this scenario involves:
- Real-time Monitoring: Continuous monitoring of the environment through sensors to detect changes.
- Pattern Recognition: Identification of patterns and trends in the dynamic events.
- Predictive Modeling: Forecasting future states based on past observations and patterns.
- Optimized Response: Determining the optimal course of action to address the detected problems and restore order, adjusting strategies based on predictions.
Challenges include unpredictable events, incomplete information, and the need for rapid decision-making.
Future Implications and Potential
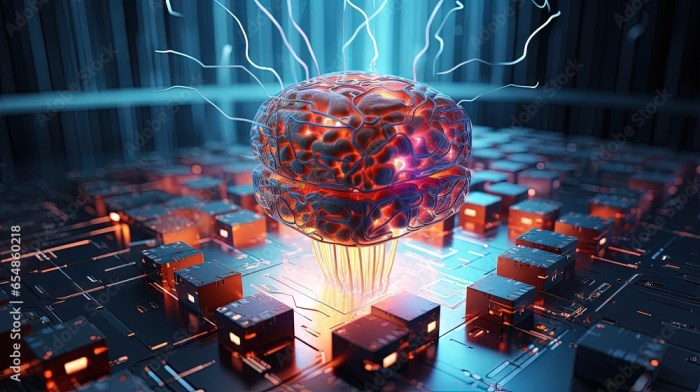
The “cat-like” cognitive computing approach, inspired by the feline brain’s remarkable abilities, promises a paradigm shift in artificial intelligence. Beyond simply replicating human-like intelligence, this research seeks to emulate the adaptability, efficiency, and intuitive problem-solving of animal cognition. This opens up exciting possibilities for various fields, from robotics to healthcare, demanding careful consideration of both potential benefits and risks.This innovative approach suggests a future where machines can interact with the world in a more nuanced and responsive way, surpassing the limitations of current AI models.
The potential applications are vast, but the journey is fraught with ethical considerations that must be addressed proactively.
Potential Future Directions and Developments
The research on cognitive computing, emulating feline cognitive processes, anticipates future advancements in several key areas. These advancements include the development of more sophisticated robotic systems capable of navigating complex environments autonomously, learning from experience, and adapting to unexpected situations. Further development may lead to more efficient and accurate medical diagnoses, personalizing treatment plans, and predicting disease outbreaks.
The field of human-computer interaction will likely experience a significant transformation, with interfaces becoming more intuitive and responsive to user needs.
Broader Societal Impact
The societal impact of this cognitive computing approach is multifaceted. Positive impacts may include enhanced efficiency in various industries, leading to economic growth and increased productivity. More advanced diagnostics and personalized treatments could lead to improvements in public health and reduced healthcare costs. However, ethical concerns, such as potential job displacement and the equitable distribution of benefits, require careful consideration and proactive measures to mitigate these risks.
Ethical Considerations
The development and deployment of “cat-like” cognitive computing systems raise critical ethical questions. Issues of algorithmic bias, data privacy, and the potential for misuse of the technology must be addressed proactively. Robust regulations and ethical guidelines are essential to ensure responsible development and deployment, preventing unintended consequences. Transparency in the decision-making processes of these systems and ongoing monitoring are vital to ensure fairness and accountability.
Potential Benefits and Risks, Ibm researchers go way beyond ai with cat like cognitive computing
Potential Benefit | Potential Risk |
---|---|
Enhanced efficiency and productivity in various sectors (e.g., manufacturing, logistics). | Potential job displacement in sectors where automation is possible. |
Improved medical diagnostics and personalized treatments, leading to better patient outcomes. | Data security breaches and misuse of sensitive patient information. |
Development of more intuitive and user-friendly human-computer interfaces. | Algorithmic bias leading to unfair or discriminatory outcomes. |
Autonomous systems capable of performing complex tasks in hazardous environments (e.g., disaster relief). | Potential misuse of autonomous systems for malicious purposes. |
Potential Advancements in Human-Computer Interaction and Communication
The “cat-like” cognitive computing approach promises to revolutionize human-computer interaction. Machines will likely become more responsive and adaptive, learning user preferences and adjusting their behavior accordingly. This could lead to more natural and intuitive interactions, enabling users to communicate more effectively and efficiently with machines. This evolution could potentially transform our daily lives, making tasks simpler and interactions more seamless.
Consider a future where everyday devices anticipate our needs, providing assistance and support without explicit instructions.
Ultimate Conclusion
In essence, IBM’s research pushes the boundaries of artificial intelligence, offering a glimpse into a future where machines can think and act more like humans. The “cat-like” cognitive computing approach, while still in its early stages, holds immense potential for revolutionizing various sectors. The exploration of these new capabilities opens doors to innovative solutions and a deeper understanding of intelligence itself.
The implications are far-reaching, and the future looks promising.